In the rapidly advancing fields of intelligent transportation and security monitoring, accurate and efficient vehicle model recognition technology has become increasingly important. Applications such as traffic flow statistics, parking lot management, and vehicle tracking in security systems impose high demands on the precision and speed of vehicle model recognition. Traditional methods often fall short due to insufficient accuracy or overly large models that reduce efficiency, making them unsuitable for practical scenarios. To address these challenges, this project explores fine-grained vehicle model recognition using Bilinear Convolutional Neural Networks (BCNN) and its improved versions. The project's innovations lie in enhancing BCNN to better extract fine-grained features for complex vehicle models and optimizing the model through innovative compression techniques to reduce its size significantly. The experimental results demonstrate that the proposed method achieves a vehicle classification accuracy of 88.68%, a high level compared to similar studies, meeting the precision requirements of various applications. Additionally, the model size is reduced by 47.4% compared to traditional methods, lowering storage and computational costs. This enables efficient operation on resource-constrained devices, laying a solid foundation for widespread application of the technology.
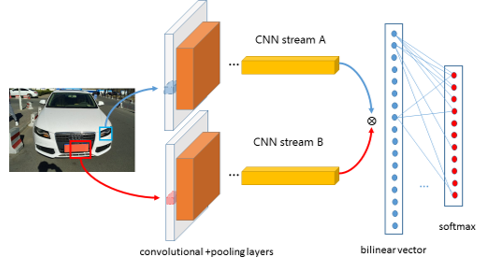